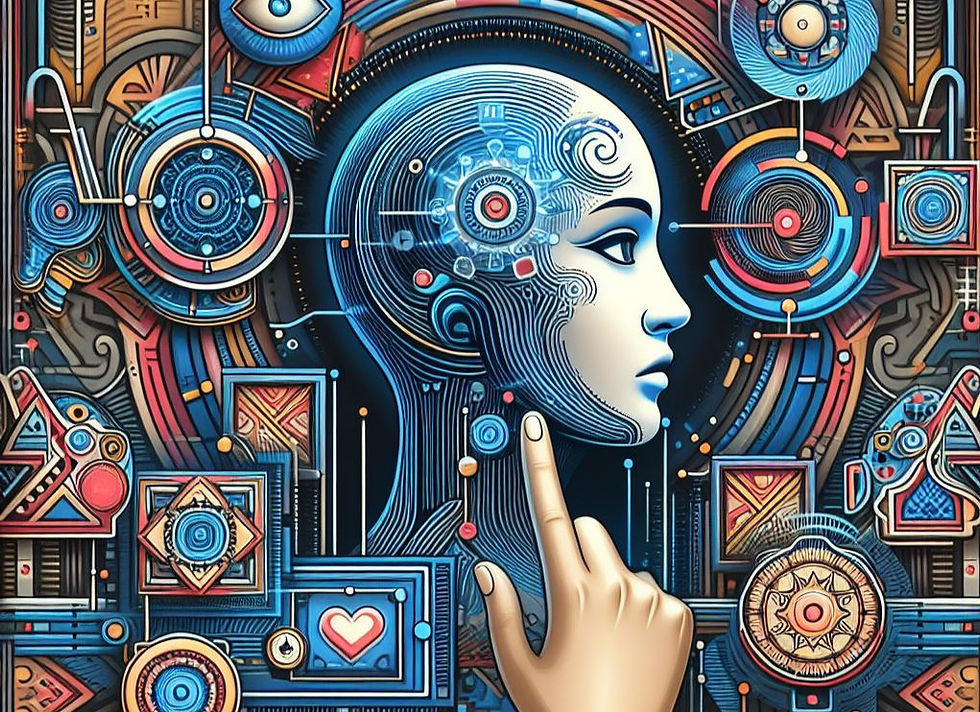
"Ethics is the anchor that keeps our AI-driven ship of insights steady in the unpredictable seas of user behaviors."
In the dynamic landscape of user research, the infusion of Artificial Intelligence (AI) has emerged as a transformative force, reshaping the way we glean insights into user behaviors and preferences. As organizations strive to deepen their understanding of users and improve digital experiences, the integration of AI has become increasingly prevalent. However, navigating this frontier requires a nuanced approach, balancing the promises of efficiency and depth with the ethical considerations and potential pitfalls associated with AI implementation.
In this article, we delve into the dos and don'ts of utilizing AI in the user research process. From leveraging AI to uncover intricate patterns in vast datasets to avoiding the pitfalls of biased algorithmic decisions, this exploration aims to provide researchers with a comprehensive guide to harnessing the power of AI for enhanced insights while maintaining ethical standards.
In recent years, the integration of Artificial Intelligence (AI) in user research has become increasingly prevalent, revolutionizing the way we understand user behaviors and preferences. While AI holds the potential to enhance the efficiency and depth of user research, it comes with its own set of challenges and ethical considerations.
Do's :
Do: Prioritize transparency when using AI in user research. Clearly communicate the role of AI in the research process and how user data will be utilized.
Example: Providing users with clear information about how AI algorithms contribute to improving their experience on a platform, fostering trust and understanding.
Leverage AI for Data Analysis:
Do: Use AI to identify patterns and uncover valuable insights from large volumes of unstructured text data, saving hours of manual effort.
Example: Employ machine learning algorithms to analyze extensive user feedback, revealing common themes and sentiments.
Use AI for Natural Language Processing (NLP):
Do: Implement NLP to transcribe and analyze interview recordings, extracting key themes and sentiments automatically.
Example: Utilize NLP algorithms to categorize and transcribe user interviews, streamlining the qualitative data analysis process.
Employ AI for Sentiment Analysis:
Do: Capitalize on AI tools equipped with sentiment analysis capabilities to identify user emotions and attitudes expressed in interviews, surveys, or social media posts.
Example: Integrate sentiment analysis algorithms to gauge user sentiments, providing nuanced insights beyond surface-level feedback.
Automate Tedious Tasks:
Do: Free up valuable time and resources by automating repetitive tasks with AI, allowing researchers to focus on more complex and strategic endeavors.
Example: Use automation tools, powered by AI, to transcribe interviews, categorize data, and handle routine tasks, enhancing overall research efficiency.
"Unlocking the secrets of user behavior requires the delicate touch of human understanding, guided by the precision of artificial intelligence."
Don'ts:
Don't: Assume that AI insights are universally applicable. Consider cultural and contextual differences in user behavior to ensure the relevance of research findings.
Example: Ignoring regional nuances identified through AI-driven research may lead to product failures in specific markets.
Don't Rely Solely on AI:
Don't: Replace human judgment with AI-generated insights. Always validate AI findings with other research methods to ensure accuracy.
Example: Relying exclusively on automated sentiment analysis without considering the context of user comments may lead to misinterpretations.
Don't Ignore Bias:
Don't: Overlook biases inherent in AI models. Be aware of potential biases and take proactive steps to mitigate them.
Example: Using an AI model trained on a specific demographic may introduce bias, affecting the accuracy of insights for a more diverse user base.
Don't Overlook Privacy Concerns:
Don't: Neglect user privacy when utilizing AI in user research. Ensure compliance with data protection regulations and prioritize user consent.
Example: Analyzing social media data without obtaining user consent may lead to privacy concerns and backlash.
Don't Use AI Without Understanding:
Don't: Implement AI without a basic understanding of how it works and its limitations. Researchers should be knowledgeable about the technology to use it effectively.
Example: Deploying AI tools without understanding their underlying principles may result in misinterpretations and flawed research outcomes.
Statistics:
According to a survey by McKinsey, organizations using AI in their customer experience strategies have reported a 10-15% increase in customer satisfaction.
A study by Gartner predicts that by 2024, 25% of customer service and support operations will integrate virtual customer assistants (VCAs) or chatbots across engagement channels, contributing to time and cost savings.
However, a report by the AI Now Institute highlights that biased algorithms in AI systems can lead to discriminatory outcomes, with women and minorities often being disproportionately affected.
"AI is the silent collaborator in the user research journey, amplifying our efforts while demanding our vigilance."
Good Examples
Chatbots for User Interviews: Chatbots for User Interviews: AI-powered chatbots have been used to conduct user interviews. They can ask predefined questions and record responses, making the process more efficient. For instance, a company might use a chatbot to gather initial user feedback on a new product feature.
AI for Sentiment Analysis: Companies like Netflix and Amazon use AI to analyze user reviews and feedback. The AI can identify common themes and sentiments, providing valuable insights that lead to product improvements.
AI in E-commerce Analytics: AI is used to analyze e-commerce data, helping companies optimize their sales funnels and customer traffic to maximize profits.
Bad Examples
Gannett’s AI Tool for Sports Articles: In 2023, newspaper chain Gannett used an AI tool called LedeAI to write sports articles. However, the articles were repetitive, poorly written, and lacked key details, leading to a negative response from readers.
iTutorGroup’s Recruiting AI: iTutorGroup, a tutoring company, used an AI tool for recruiting that rejected applicants based on their age. This led to a lawsuit and a settlement of $365,000.
Lack of AI Transparency: AI decisions are not always intelligible to humans, leading to a lack of transparency. This can result in biased or unsafe decisions, as the data AI algorithms use may not be clear.
"AI is the ally, not the oracle, in the quest for understanding users. The questions we ask and the context we consider remain our true north."
Suggested processes and steps that a user researcher can take to enhance their daily tasks and improve their outputs:
Integrate AI for Data Analysis:
Process: Incorporate AI tools for data analysis, allowing for a more efficient identification of patterns and trends in user feedback.
Steps:
Identify areas in your research where AI can assist in uncovering insights from large datasets.
Research and implement AI-driven analytics tools that align with your research goals.
Regularly update and fine-tune AI algorithms to ensure accuracy and relevance.
Automate Repetitive Tasks:
Process: Streamline your workflow by automating repetitive and time-consuming tasks using AI.
Steps:
Identify tasks that are repetitive and can be automated, such as transcribing interviews or sorting qualitative data.
Explore AI-powered tools designed for automation and integrate them into your research process.
Monitor and evaluate the performance of automated processes to ensure accuracy.
Implement AI for Personalization:
Process: Enhance user experiences by utilizing AI to analyze individual behaviors and preferences for personalized insights.
Steps:
Identify touchpoints where personalized user experiences can be implemented, such as in product recommendations or content delivery.
Choose or develop AI algorithms that align with the goals of personalization without compromising user privacy.
Regularly assess and refine personalization strategies based on user feedback and changing preferences.
Predict User Behavior with AI:
Process: Proactively adjust interfaces or content by leveraging AI to predict user behavior and preferences.
Steps:
Implement AI models that can predict user actions, such as click-through rates or engagement levels.
Monitor the accuracy of predictions and adjust models based on real-time user feedback.
Collaborate with UX/UI designers to implement changes based on AI-driven insights.
Enhance Accessibility with AI:
Process: Improve accessibility by incorporating AI features like speech recognition and text-to-speech capabilities.
Steps:
Identify areas in your digital platforms where accessibility features can be enhanced.
Research and implement AI-driven accessibility solutions, ensuring they align with industry standards.
Conduct user testing with individuals who have accessibility needs to validate the effectiveness of AI enhancements.
Avoid Overreliance and Bias:
Process: Maintain a balance between AI and human insights, while actively mitigating biases in AI algorithms.
Steps:
Regularly cross-verify AI-generated insights with traditional research methods to ensure accuracy.
Stay informed about biases in AI models and proactively address them through continuous monitoring and updates.
Conduct diversity audits to assess the representation of different user groups in your research data.
Prioritize Transparency and Ethical Considerations:
Process: Communicate clearly with users about the role of AI in the research process and prioritize ethical considerations.
Steps:
Develop clear communication strategies to inform users about the use of AI and how their data will be handled.
Establish and follow ethical guidelines for user research involving AI, including obtaining informed consent.
Regularly review and update privacy policies to align with evolving industry standards.
By incorporating these processes and steps into their daily tasks, user researchers can harness the power of AI while ensuring transparency, ethical practices, and improved overall research outcomes. User researchers play a crucial role in understanding user needs, behaviors, and motivations to inform the design process. Their daily tasks often involve conducting interviews, surveys, and usability tests, analyzing data, and presenting findings to stakeholders.
Here are some steps that user researchers can take to improve their outputs when using AI:
Understand AI Capabilities: Gain a basic understanding of how AI works, its capabilities, and limitations. This will help you leverage AI tools effectively and responsibly.
Combine AI with Traditional Methods: Use AI to complement, not replace, traditional research methods. For example, you can use AI for initial data analysis and then validate the findings with in-depth interviews or focus groups.
Check for Bias: Always be aware of potential bias in AI models. Regularly review and update your AI tools to ensure they are trained on diverse and representative data.
Respect User Privacy: Ensure that you are complying with all relevant data protection regulations when using AI in user research. Be transparent with users about how their data will be used.
Continual Learning and Improvement: Keep up-to-date with the latest developments in AI and user research. Regularly review and refine your processes to ensure you are using AI in the most effective and ethical way.
By following these steps, user researchers can harness the power of AI to enhance their research and deliver more valuable insights. Remember, the goal is to use AI as a tool to aid user research, not as a replacement for human judgment and understanding.
Conclusion:
As we conclude our exploration of AI's role in user research, it becomes evident that the successful integration of AI demands a delicate balance between innovation and responsibility. The dos, ranging from augmenting analysis with AI to enhancing accessibility, illuminate the vast potential AI holds for revolutionizing user research. These strategies, when implemented thoughtfully, empower researchers to uncover deeper insights, automate tedious tasks, and predict user behaviors.
On the flip side, the don'ts underscore the importance of avoiding overreliance on AI, addressing biases, and prioritizing ethical considerations and transparency.
By embracing the suggested processes and steps, user researchers can embark on a journey where AI serves as a powerful ally in uncovering user insights while upholding the principles of fairness, transparency, and user privacy.
In the ever-evolving realm of user research, the synergy between human expertise and AI capabilities is not just a choice but a strategic imperative for unlocking the full potential of digital experiences.
AI holds immense potential to enhance user research by automating tedious tasks, providing valuable insights, and streamlining processes. However, it’s crucial to use it responsibly and be aware of potential pitfalls such as bias and privacy concerns. By understanding AI’s capabilities and limitations, combining it with traditional research methods, checking for bias, respecting user privacy, and continually learning and improving, user researchers can harness the power of AI to deliver more valuable insights. Remember, the goal is not to replace human judgment and understanding with AI, but to use it as a powerful tool to aid user research. As we continue to explore the possibilities of AI in user research, let’s strive to do so with responsibility, transparency, and a commitment to ethical practices. Happy researching!
Article by Mr.Tushar Deshmukh, CEO & Founder UXExpert, Dir. UXUITraining Lab Pvt. Ltd. other services - UXResearch, UXUIHiring, UXTalks, UXTools
UXExpert - is India's top 10 User Experience Service Provider.
UXUITraining Lab Pvt. Ltd. is India's top mentoring and training provider for User Experience
UXTalks Interviews, talk shows, events Live talk show where we discuss about Design

Comments